RESEARCH: SSI-recognized research places third at MIT Sloan Sports Analytics Conference
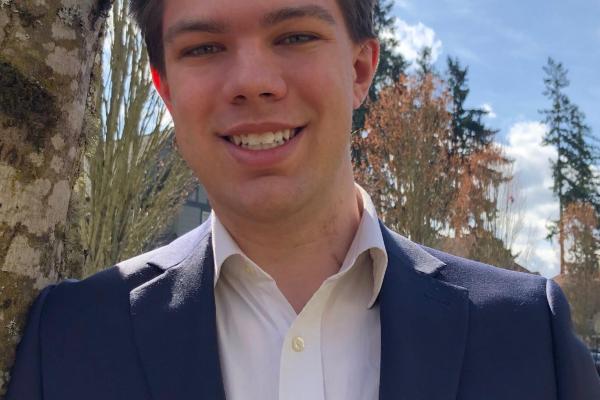
Economics majors Alexander Williams (pictured), Seth Brugler and Ben Clarke, former winners of the SSI Sports Analytics Grant, finished third in the MIT Sloan Sports Analytics Conference research paper competition.
This is a phenomenal achievement, as they were competing against some of the best sports analytics researchers-- at all ages and levels of experience-- from around the world.
Their paper was entitled "MAYFIELD: Machine Learning Algorithm for Yearly Forecasting Indicators and Estimation of Long-Run Player Development" and their project has been previously presented and nurtured as part of the annual SSI Undergraduate Research Fairs and the 2020 SSI Sports Analytics Conference.
Here is a summary of their research:
Abstract
Accurate statistical prediction of American football player development and performance is an important issue in the sports industry. We propose and implement a novel, fast, approximate k-nearest neighbor regression model utilizing locality-sensitive hashing in highly dimensional spaces for prediction of yearly National Football League player statistics. MAYFIELD accepts quantitative and qualitative input data, and can be calibrated according to a variety of parameters. Concurrently, we propose several new computational metrics for empirical player comparison and evaluation in American football, including a weighted inverse-distance similarity score, stadium and league factors, and NCAA-NFL statistical translations. We utilize a training set of comprehensive NFL statistics from 1970-2019, across all player positions and conduct validation on the model with the subset of 2010-19 NFL statistics. Preliminary results indicate the model to significantly improve on current, publicly available predictive methods. Future training with advanced statistical datasets and integration with scouting-based methods could improve MAYFIELD's accuracy even further.